Bioinformatics and Sensory Function of Mammalian Fur: An Analysis of Coat Patterning, Whiskers, and Trigger Hairs
Marc Amin, Lovéni Hanumunthadu, Matthew MacDonald, Thibaud Roy
Introduction
The Turing pattern, which is further explained in the paper, is an observed pattern in natural systems ranging from cheetah print to coral growth. Alan Turing proposed a mathematical model to describe pattern formation based on reaction and diffusion rates of morphogens, and this model holds true for a vast number of biological systems. A morphogen is a substance that controls tissue and pattern development, and Turing's model uses the diffusion and composition of those morphogens to give a seemingly random pattern distribution predictability in nature.
The specialization of hair as whiskers is seen in a wide variety of mammals. Evolved from hair, whiskers provide sensory information to the animal through mechanoreceptors located in the base of the hair follicle. The complexity of this sensory function can be simplified to mechanical data being transformed into useful conclusions about the animal's environment. Every rotation and axial force on a whisker are used to create a continuous “image” of spatial properties. Interestingly enough, the distribution of a rat's whiskers on its face follows a Turing pattern. The curvature of a whisker can also be modeled by a Euler function.
A comparison can be made with Trap deployment in carnivorous plants, which follows a similar sensory pathway to whiskers. Both use mechanical input to elicit a neural response. In Venus fly traps, mechanosensitive ion channels transmit an electrical signal upon disturbance, much like the action potential of whiskers.
These patterns and sensory systems are both ways for us to interpret and appreciate the complexity of nature's systems in a format we can understand and quantify.
Reaction-Diffusion Systems
In 1952, the English mathematician and computer scientist, Alan Turing, proposed an approach (an “algorithm”) to explain biological pattern formation occurring in nature (Green and Sharpe, 2015). Turing hypothesized a process called morphogenesis to explain these patterns, and he defined two types of morphogenesis: mechanical and chemical (Turing, 1952). The mechanical part is described by the velocities, masses, positions, elastic properties, and the forces of the morphogens at play, while the chemical approach is described by the composition and the diffusibility of the morphogens (Turing, 1952). However, being the biologist he was, Turing's research mostly discussed chemical morphogenesis, which is favorable for this paper.
According to Turing, natural patterns can be produced in space and time by two chemical processes: chemical reactions and chemical diffusion. Reaction is the chemical process where a substance is changed to another substance (reactants to products) while diffusion is the process where a substance spreads from a milieu of high concentration to one with lower concentration, following a concentration gradient. His formula states:
∂_t q =Ḏ ∇^2 q+R(q)
(1)
where, q(x,t) is the unknown vector function, D is the diagonal matrix of diffusion constants, and R is the reaction function for q (Eq. 1) (Turing, 1952). To simplify, we can write:
{∂u\over ∂t}=D_u {(∂^2 u)\over (∂x^2 )}+f(u)
(2)
where Du (∂2u/∂x2) is the diffusion component, f(u) is the reaction component, and Du is the diffusion constant (Eq. 2; “7. Turing Instabilities and Coat Patterns” | TheShapeofMath | YouTube, 2013). In 1972, Gierer and Meinhardt generalized Turing's idea to produce a Gierer Meinhardt Model, derived from Brownian motion and Turing's equation (Gierer and Meinhardt, 1972):
{∂u\over ∂t}=D_u {(∂^2 u)\over (∂x^2 )}+{u^2\over v}-bu
(3)
{∂u\over ∂t}=D_u {(∂^2 u)\over (∂x^2 )}+u^2-v
(4)
where u is the activator and v is the inhibitor. To produce stable Turing patterns, Dv should be larger than Du (Green and Sharpe, 2015).
Visualizing Eq. 3 and Eq. 4, and respecting that an activator has short-range of diffusion and the inhibitor has long-range of diffusion, we can plot a relative diagram that shows the reaction-diffusion system (Green and Sharpe, 2015) (Fig. 1).
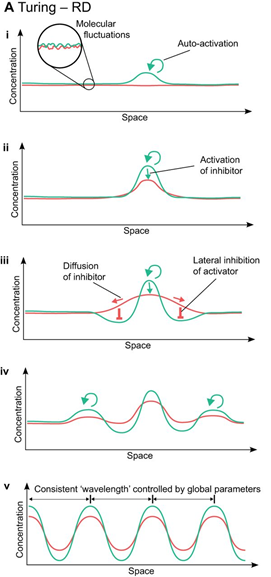
At the start, the activator concentration rises, which starts to boost the production of inhibitors (Fig. 1i and ii). Hence, the inhibitor starts to suppress the production of activators. However, due to the inhibitor having faster diffusion, it is capable of suppressing activators away from the peak (iii). By having inhibition near the middle peak, the activators are repressed and cannot peak near the origin (iv). By repeating this process multiple times, we end up with a consistent pattern that explains the reaction-diffusion system.
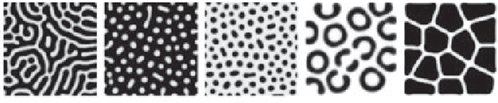
Turing patterns can come in an array of shapes and forms (Fig. 2). For example, if an activator has a high diffusion rate, but still lower than the inhibitor's diffusion rate, it can create labyrinth patterns because the activators areas will merge with neighboring activator areas by simply diffusing. This is known to be as the activator is leaking (Rivera, 2020).
Naturally occurring examples of Turing patterns can be seen in the labyrinth-like patterns of brain coral, the scales pattern found on different fish species, and even the seed distribution in flowers. More specific on our subject, examples of these patterns can be seen in hair follicle and hair pigmentation distribution, like stripes on zebras and spots on leopards, which may seem random at first, but follow a reaction-diffusion system. Below, we will explore the intriguing patterns found on Cheetah fur coats (“Can Math Explain How Animals Get Their Patterns?” | MinuteEarth | YouTube, 2016).
Cheetah Fur Coat Pigmentation
Before exploring the reaction-diffusion systems that explain how Acinonyx jubatus, also known as the cheetah, develop spots, stripes and spot-to-stripe transitions (Fig. 3), it is important to discuss how cheetahs, and mammals in general, develop hair follicle distribution.
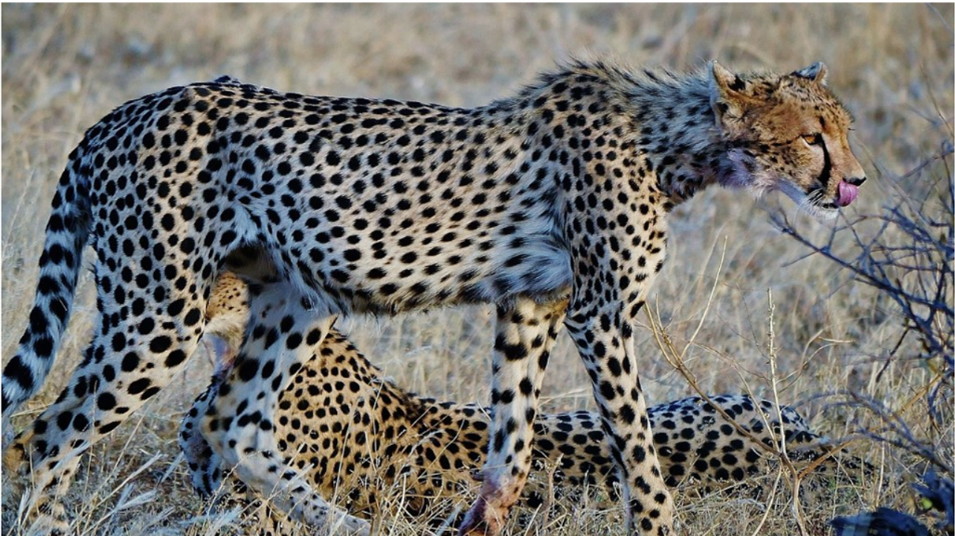
Although fur distribution on the skin may seem random at first sight, these hair follicles are regulated by a Turing pattern, caused by a reaction-diffusion mechanism in the epidermis (Hiscock and Megason, 2015). Following the same Gierer Meinhardt model established by Eq.3 and Eq. 4 to provide an activator and inhibitor model, the same patterning can be seen in mammalian coats. One pair of activator and inhibitor that researchers think are responsible for coat patterning are Wnt and Dkk proteins respectively (Hiscock and Megason, 2015). On one hand, the activator Wnt reacts to activate the production itself and Dkk, while diffusing slowly. On the other hand, the inhibitor Dkk rapidly diffuses and reacts to inhibit the production of Wnt (Fig. 4). These diffusion rates guarantee local activation, long-range inhibition, which creates the Turing distribution that can be seen. However, researchers are not certain if this pair is responsible for the entire process, or if it is cooperating with other patterning mechanisms (Hiscock and Megason, 2015).
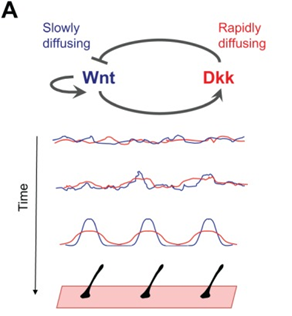
Some researchers dismiss the fact that Wnt/Dkk are the actual activator/inhibitor pair and suggest alternatively that Edar/BMP are the actual morphogens at work (Mou et al., 2006). Ectodysplasin receptor (Edar) and bone morphogenetic protein (BMP) may be the morphogens responsible for fur coat distribution, but more research is leaning towards the Wnt/Dkk pair.
Now that we have established a basis on how fur distribution generally occurs on the mammalian body, it is only reasonable to discuss how cheetahs develop two different types of fur pigmentation. In general, mammals have two types of melanin that controls pigmentation in fur: pheomelanin, which creates a yellow tint in fur, and eumelanin, which creates the darker tints (Jackson, 2013). To produce both types of melanin, felidae, the cat family which cheetahs are a part of, have a highly regulated process that can have consequences on the fur color that is displayed in a certain region on the coat. In the DNA, the expression of the agouti gene during hair growth blocks the receptor melanocortin 1 (MC1R), which promotes the synthesis of pheomelanin by preventing eumelanin from being expressed. For the synthesis of eumelanin, melanocyte-specific genes, like tyrosinase, Dct and Tyrp1, are known to be activated (Jackson, 2013). However, these melanocyte-specific genes have no change on agouti expression. Instead, researchers have found that, alternatively, there is an increase in the expression of endothelin-3 (Edn3), which is the ligand of endothelin receptor B, responsible for melanocyte proliferation and differentiation during hair development. Accordingly, an increased expression of Edn3 correlates with the presence of eumelanin, making the fur in these areas darker (Jackson, 2013) (Fig. 5b).
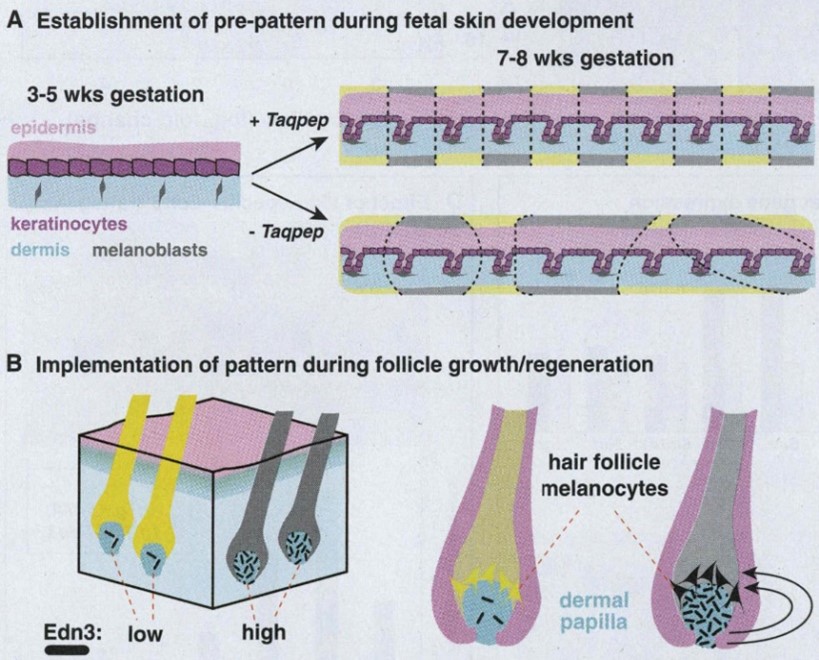
Another gene that affects the coat pattern in Felidae is one that expresses Transmembrane aminopeptidase Q (Taqpep), which is mutated in cheetah's that have blotches, also known as a king cheetah (Kaelin et al., 2012) (Fig. 5a). An unmutated allele allows the spots to form normally. Not much is known about the origin of this allele, but its mutation occurs as a frameshift insertion from the genotype of the cheetah to the one of the king cheetahs (Fig. 6). This mutation, not being too drastic for the king cheetah, simply makes the spots that would naturally occur in a normal cheetah diffuse into neighboring spots. By spreading out, the spots become lines, and end up blotching into either stripes or irregular shapes (Fig. 5a). A parallel can be made here with Turing patterns, since the mutated Taqpep allows spots to spread compared to non-mutated Taqpep. Hence, instead of forming normal spots, the pattern becomes a labyrinth-like pattern (refer to Fig. 2). In this analogy, Taqpep could be considered an activator that has a faster than usual diffusion rate, allowing it to make the spots leak (Kaelin et al., 2012).
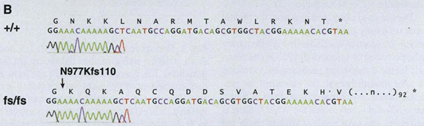
Hence, there are two main proteins that can affect the overall pattern of the cheetah coat:
Transmembrane aminopeptidase Q (Taqpep) and Endothelin-3 (Edn3) (Kaelin et al., 2012). Combining the Turing “behaviors” of hair follicle distribution and hair pigmentation, the result shows how cheetah coats have the default spots pattern. However, as mentioned earlier, cheetahs also have stripes on their tails. The reasoning behind this transition is that the tail of the cheetah is long and narrow. When reaction-diffusion occurs in thin areas, there is only one direction where diffusion can occur, which converts a “natural spots” pattern into a stripe pattern. There are three more reasons why spots can transition to stripes: production gradient, where expression of both activator and inhibitor are increased at one end of the tissue; parameter gradient, where a protein or cofactor binds to an activator and prevents it from activating at one end of the tissue; and directional growth, where the tissue grows directionally, like for digit formation (Rivera, 2020). However, only limited diffusion causes cheetah tails to develop stripes.
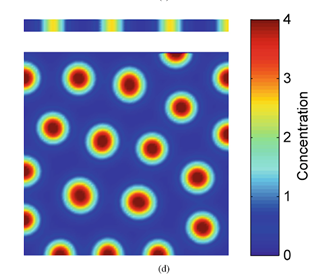
on the bottom shows spot formation when the vertical height of the area is increased (“7. Turing Instabilities and Coat Patterns” | TheShapeofMath | YouTube, 2013).
Following the reasoning established above, we can assume that cheetahs, or any feline in that matter, having wider tails will have their spots distribution reach further down the distal end of their tails. However, cheetahs that have thinner tails will have their spot distribution end closer to the proximal end of the tails, allowing them to have more stripes on their tails (Rivera, 2020).
Whiskers
Facial vibrissae, otherwise known as whiskers, are a common trait in almost every living mammal on Earth today. Whiskers may have been some of the first hairs to evolve in mammalian ancestors, even before hair responsible for thermoregulation appeared (Grant et al., 2013). Whiskers are specialized hairs that are characterized as long length, well-innervated, and connected to the somatosensory cortex of the brain. The complex sensory capabilities of whiskers can be related very simply to data collection and interpretation. While obviously a very simplified analogy, thinking of a whisker as a tool for data collection allows us to think of the brain and nervous system as a computer that interprets this data to provide the animal with useful information about the space it occupies. In rats, this system is called the vibrissae sensory system. Due to the abundant research on this system, rats will be the primary focus in this section.
Whiskers collect mechanical data from the environment, and with that data different conclusions can be drawn about the animal's surroundings. An important distinction to be made about whiskers is that spatial data is accumulated over time, not just on tactile snapshots (Fassihi et al., 2020). The continuous collection of spatial data allows for more accurate conclusions about texture and pattern recognition (Fassihi et al., 2020). Mechanoreceptors lie at the base of the whisker in the hair follicle. There, forces applied on the whisker are transduced by the mechanoreceptors and there is a resultant action potential. The mechanoreceptor is a collection of nerve endings that measure the deformation of tissue in the whisker due to external stimuli. Interestingly, the diversity of nerve endings in the mechanoreceptor may indicate that they are specialized in detecting various physical stimuli (Petersen, 2007). In rats, mechanical information is conveyed to the trigeminal ganglion (Tonomura et al., 2015). Located at the base of the skull, this collection of neurons interprets the action potentials induced by mechanoreceptors (Kwegyir-Afful et al., 2008). From the trigeminal ganglion, sensory information is sent to the thalamus. Another synapse is then projected to the primary barrel cortex from the thalamus (Petersen, 2007). This pathway is visualized in Fig. 8.
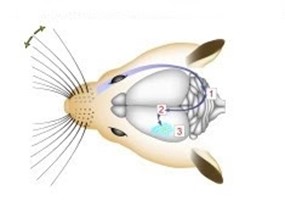
The barrel cortex is responsible for the interpretation of mechanical data, and does so by generating associations between specific sensory input like texture, shape, etc. (Petersen, 2007). This process falls under the category of neural coding, which is a broad description for the cooperative activity of neurons in the brain (Richmond, 2001). The distribution of whiskers on the face of a rat is almost identical to the distribution of nodes within the barrel cortex, as seen in Fig. 9.
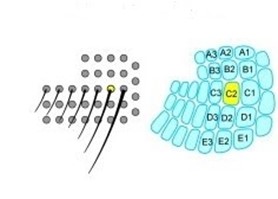
An advantage of whiskers is their simplicity – mechanically, they can be reduced to a beam. Whiskers are typically 10 times as long as their base (Campagner et al., 2018). A variety of stimuli are collected by mechanoreceptors, namely axial/lateral forces, bending moments, and even inertia (Campagner et al., 2018). Whiskers have variable stiffness along their body (Campagner et al., 2018). Because mechanoreceptors lie at the base of the whisker, motion at the base is more relevant to neural coding than motion at the tip (Tonomura et al., 2015). There are both kinematic variables (velocity, position, etc.) and mechanical forces acting on the whisker at any given time, however it is ultimately the mechanical forces that determine the corresponding neural response (Campagner et al., 2018). In theory, six total mechanical variables in three dimensions can be applied to the whisker at one time, with an applied force as well as a moment of the base of the whisker. In a steady state, these forces will have their counterparts to keep the whisker in equilibrium (Campagner et al., 2018). However, it has been observed that when a rat's whisker is subject to external stimuli in uncontrolled settings, whisking motion mostly happens in a horizontal plane (Campagner et al., 2018). This can be rationalized by simply thinking of how a rat would interact in nature, seldom moving on any plane other than horizontal.
Whisker Curvature
As discussed in the section above, whiskers are a powerful sensory tool for mammals, and many terrestrial animals, such as rats, will undergo “whisking”, in which their vibrissae will bend over objects, helping the mammal make fine distinctions of texture (Arabzadeh et al., 2018). The connection between whisker curvature and the ability to sense its environment are interlinked.
Rats make for interesting exploration of the connection between whiskers and somatosensory cortex because of the one-to-one somatotopic mapping of whiskers to the barrel cortex, meaning that each whisker is represented by a well-defined section of the rat's brain (Hobbs et al., 2015). Therefore, there may be a link between whisker's geometrical properties and its relation to the somatosensory cortex (Arabzadeh et al., 2018). Approximating the long, thin, rat whiskers as a centerline, a model can be made of the whisker's curvature. Using a dataset of 523 whiskers of Rattus norvegicus, a two-parameter linear curvature function can be created that corresponds to an interval of a universal Euler spiral, shown in Fig. 10.
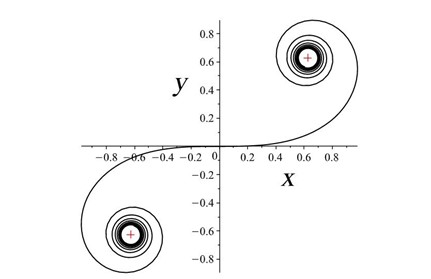
This two-parameter linear curvature function is modeled as follows. Integrating Cesàro's equations under the assumptions that all centerlines are scaled to L, the following models are made (Starostin et al., 2020):
x̂(ŝ)=A(C(Aŝ+BA)cos(B22A)+S(Aŝ+BA)sin(B22A))
(5)
ŷ(ŝ)=A(S(Aŝ+BA)sin(B22A)-(C(Aŝ+BA)cos(B22A))
(6)
where S and C are integrated Fresnel integrals, ŝ is the relative arc length, A and B are Cesàro's coefficients (Eq. 5 and Eq. 6). Using this model, mouse whiskers can be conformally mapped to the Euler spiral as shown in Fig. 11 (Starostin et al., 2020).
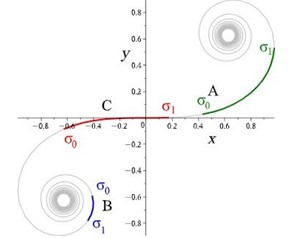
While it is not yet known what the role it plays is, there is a connection between the curvature of the rat's whiskers and its vibrissae system. The Euler spiral model plays a critical role in understanding whisker shapes and their function as sensory tools for mammalian species (Hobbs et al., 2015).
Sensory Functions
Mammalian hairs projecting from hair follicles in the skin can sense changes in the environment, such as an insect walking along the hand, thanks to sensory innervation by the hair root plexus (“Hair” | Biology for Majors II | Lumen Waymaker, n.d.). The root plexus consists of sensory nerve endings surrounding the base of the hair follicle and serves as a sensitive mechanoreceptor for touch (“Hair” | Biology for Majors II | Lumen Waymaker, n.d.; Wikipedia, 2018) (Fig. 12). When the hair root plexus senses movement of the hair at the surface of the skin, it transmits the information to the central nervous system (brain and spinal cord), which can then respond by activating the skeletal muscles of the eyes to see the insect and the skeletal muscles of the body to act against it (“Hair” | Biology for Majors II | Lumen Waymaker, n.d.).
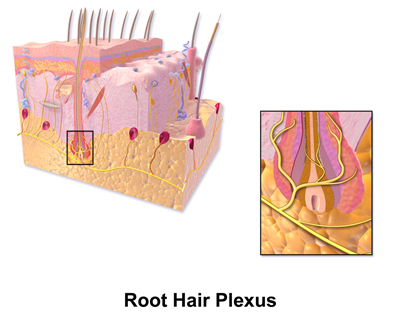
Similarly, carnivorous plants also contain sensitive hair-like structures which are required to perform a specific function, that is, trap deployment. This allows us to have more insight on how mammalian fur responds to different sensory stimuli. Carnivorous plants have five basic trapping mechanisms: snap, flypaper, pitfall, lobsterpot, and bladder traps (Editors of Encyclopedia Britannica, “carnivorous plant”). A common example of an insectivorous plant with snap trap is the Venus flytrap, also known as the Dionaea muscipula (Volkov et al., 2008).
Venus flytraps detect moving insects via highly sensitive, action potential producing trigger hairs; these mechano-sensors act as high-sensitivity levers, crucial for prey capture and digestion (Scherzer et al., 2019). As seen in Fig. 13, Dionaea trigger hairs are essential for trap closure. In an experiment with an ant, the surface potential was recorded for both wild-type Dionaea traps and those with their hairs removed; it was found out that while the hair-containing wild-type traps produced surface potential shifts, those without trigger hairs showed no change (Scherzer et al., 2019).
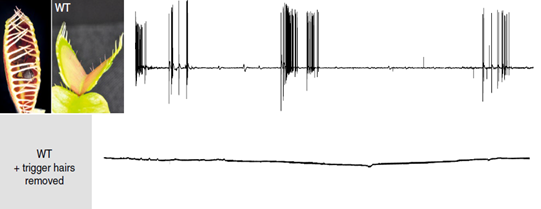
Venus flytraps can react to mechanical stimuli through mechanosensitive ion channels (Volkov et al., 2008). These sensory ion channels are activated by mechanical stress and transduce the sensed information into electrical signals, analogously to transmission of information to the central nervous system from the root plexus (Volkov et al., 2008). The Venus flytrap accumulates small subthreshold charges, and when the threshold value is reached, the trap closes (Volkov et al., 2008). Repeated application of smaller electric charges demonstrates the summation of stimuli; the cumulative character of electrical stimuli hence indicates the existence of electrical memory in this plant (Volkov et al., 2008). Fig. 14 clearly illustrates the part played by the accumulation of electric charges in the mechanism of trap deployment.
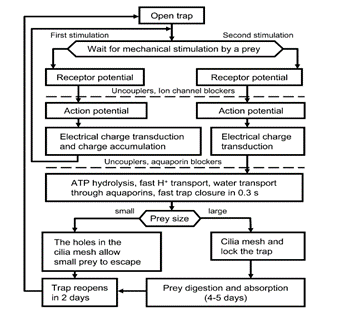
The rapid trap deployment of the Dionaea traps involves a change in the leaf geometry and can be explained by a loss of turgor in the upper epidermis, a sudden acid-induced wall loosening of motor cells, or an expansion of the cell wall through acid growth (Volkov et al., 2008). A rapid decrease in pH could also be the causing factor; traps have been shown to close when immersed in solutions to a pH of 4.5 and below, while leaves infiltrated with neutral buffers keeping the pH above 4.5 do not close in response to stimulation of their trigger hairs although APs are generated (Volkov et al., 2008).
Potential Engineering Applications
The sensory functions of whiskers in particular are very interestingly designed. The simplicity of simply pressure sensors in the tissue give rise to very complex and accurate spatial awareness, which is a design feat. The principle of inputting basic data to produce something elegant is obviously very desirable for any algorithm, but through evolution, this system has been optimized in the brain of a rat, of all things. As has been the analogy throughout the paper, creating software that mimics that of nature's own algorithms is very interesting and deserves more research. Modeling biological patterns, as done by Turing, could prove beneficial to the creation of our own systems. Nature has had millions of years to optimize their own systems and using the tried-and-true design properties of biological systems in our own design could prove beneficial.
Conclusion
Looking at the Zebra's stripes or the Nautilus shell's logarithmic growth, it is easy to visualize that distinct and similar patterns exist in nature. These patterns in nature can be quantified and mathematics can be used to illustrate some of these universal patterns. From reaction-diffusion systems to sensory information, models can be made to describe the natural world.
The variety of forms that reaction-diffusion systems can take, from spot-like to horseshoe-esque, is based on the diffusion rates of the activator or inhibitor which can be modeled using Turing's equations. An interesting example of this is the diffusion rates of the Wnt and Dkk proteins that guarantee local activation and long-range inhibition, potentially responsible for the Turing pattern seen by hair distribution on mammalian skin.
Models can also be made to describe whiskers, specifically that of rats, and their connection to the somatosensory cortex. The ends of whiskers relay important information via action potentials induced by mechanoreceptors. Additionally, the model of the curvature of whiskers as a Euler spiral is an interesting indication of the role that whiskers play as tactile sensors.
Furthermore, mammalian fur uses sensory innervation by the root plexus to sense environmental disturbances and produce feedback. This process is analogous to trap deployment in carnivorous plants: when their trigger hairs are stimulated, mechanosensitive ion channels are activated and convert the stimuli into an electric charge which can then be used to induce trap deployment by changing the conformation of the leaves.
In conclusion, the natural world is made up of interesting models once quantified, and the applications of the quantification of nature such as modeling of biological structures, is abundant.
Appendix
“Can Math Explain How Animals Get Their Patterns?.”, 11 August 2016, MinuteEarth, YouTube, https://www.youtube.com/watch?v=alH3yc6tX98.
“7. Turing Instabilities and Coat Patterns.”, 12 April 2013, TheShapeofMath, YouTube, https://www.youtube.com/watch?v=No6LvBBmk5E.
References
Arabzadeh, E., Zorzin, E., & Diamond, M. E. (2005). Neuronal Encoding of Texture in the Whisker Sensory Pathway. PLoS Biology, 3(1), e17. doi:10.1371/journal.pbio.0030017
Blaus, B. (2014). Root Hair Plexus. In (Vol. 2500 × 2000). Retrieved from https://en.wikiversity.org/wiki/WikiJournal_of_Medicine/Medical_gallery_of_Blausen_Medical_2014
Britannica, E. o. E. (2020, 9 Jul 2021). carnivorous plant. Retrieved from https://www.britannica.com/plant/carnivorous-plant
Campagner, D., Evans, M. H., Loft, M. S. E., & Petersen, R. S. (2018). What the Whiskers Tell the Brain. Barrel Cortex Function, 368, 95-108. doi:10.1016/j.neuroscience.2017.08.005
Fassihi, A., Zuo, Y., & Diamond, M. E. (2020). Making sense of sensory evidence in the rat whisker system. Neurobiology of Behavior, 60, 76-83. doi:10.1016/j.conb.2019.11.012
Gierer, A., & Meinhardt, H. (1972). A theory of biological pattern formation. Kybernetik, 12(1), 30-39. doi:10.1007/BF00289234
Grant, R. A., Haidarliu, S., Kennerley, N. J., & Prescott, T. J. (2013). The evolution of active vibrissal sensing in mammals: evidence from vibrissal musculature and function in the marsupial opossum Monodelphis domestica. Journal of Experimental Biology, 216(18), 3483-3494. doi:10.1242/jeb.087452
Green, J. B. A., & Sharpe, J. (2015). Positional information and reaction-diffusion: two big ideas in developmental biology combine. Development, 142(7), 1203-1211. doi:10.1242/dev.114991
Hiscock, T. W., & Megason, S. G. (2015). Mathematically guided approaches to distinguish models of periodic patterning. Development, 142(3), 409-419. doi:10.1242/dev.107441
Hobbs, J. A., Towal, R. B., & Hartmann, M. J. (2015). Probability distributions of whisker-surface contact: quantifying elements of the rat vibrissotactile natural scene. Journal of Experimental Biology, 218(Pt 16), 2551-2562. doi:10.1242/jeb.116186
Jackson, I. J. (2013). How the leopard gets its spots: a transmembrane peptidase specifies feline pigmentation patterns. Pigment Cell & Melanoma Research, 26(4), 438-439. doi:https://doi.org/10.1111/pcmr.12101
Kaelin, C. B., Xu, X., Hong, L. Z., David, V. A., McGowan, K. A., Schmidt-Küntzel, A., . . . Menotti-Raymond, M. (2012). Specifying and Sustaining Pigmentation Patterns in Domestic and Wild Cats. Science, 337(6101), 1536-1541. Retrieved from https://science.sciencemag.org/content/sci/337/6101/1536.full.pdf
Kwegyir-Afful, E. E., Marella, S., & Simons, D. J. (2008). Response properties of mouse trigeminal ganglion neurons. Somatosensory & Motor Research, 25(4), 209-221. doi:10.1080/08990220802467612
Maini, P. K., & Woolley, T. E. (2019). The Turing Model for Biological Pattern Formation. In A. Bianchi, T. Hillen, M. A. Lewis, & Y. Yi (Eds.), The Dynamics of Biological Systems (pp. 189-204). Cham: Springer International Publishing. doi: https://doi.org/10.1007/978-3-030-22583-4_7
Metz, H. C., Manceau, M., & Hoekstra, H. E. (2011). Turing patterns: how the fish got its spots. Pigment Cell & Melanoma Research, 24(1), 12-14. doi:https://doi.org/10.1111/j.1755-148X.2010.00814.x
Mou, C., Jackson, B., Schneider, P., Overbeek, P. A., & Headon, D. J. (2006). Generation of the primary hair follicle pattern. Proceedings of the National Academy of Sciences, 103(24), 9075. doi:10.1073/pnas.0600825103
Petersen, C. C. H. (2007). The Functional Organization of the Barrel Cortex. Neuron, 56(2), 339-355. doi:10.1016/j.neuron.2007.09.017
Rivera, A. (2020). Turing Patterns to Generate Stripes and Spots. In Evolutionary Developmental Biology (Rivera). Retrieved from https://bio.libretexts.org/@go/page/14885
Roehl, S. (2015). Cheetah at Samburu. In (pp. Young Cheetah half an hour after feeding on an impala youngster. The mother is just behind it on the ground.). Flickr. Retrieved from https://www.flickr.com/photos/42964440@N08/26437674936/in/photolist-E21DK2-RYwmYj-AimWFm-PP2zwJ-DxLzSe-xqhJq6-GkZDaQ-RvQDom-FV9UPY-H1z8wy-Aixa3M-Gep9ie-Fim4DT-MuxkZH-rSAHnb-MAgCGi-WPRfM6-tJEQFb-Rrr266-CFiipu-REEAYe-DYBYMw-23emCgw-oCXbrk-F1x4EK-Nne5sF-biKRft-Ps9URp-YiTYs8-NVcgSJ-ukCUm9-EU28Kq-GhcZFE-CQfC18-Gk1qUX-HTMC57-FiXbFu-Y5tuhq-P4UTGA-SjEctH-PQTkiy-yeX6mn-U9genx-5pvr48-tFupN3-P2rukq-GpyPYe-5jy5tB-P7fRQn-NBZqBg
Scherzer, S., Federle, W., Al-Rasheid, K. A. S., & Hedrich, R. (2019). Venus flytrap trigger hairs are micronewton mechano-sensors that can detect small insect prey. Nature Plants, 5(7), 670-675. doi:10.1038/s41477-019-0465-1
Starostin, E. L., Grant, R. A., Dougill, G., van der Heijden, G. H. M., & Goss, V. G. A. (2020). The Euler spiral of rat whiskers. Science Advances, 6(3), eaax5145. Retrieved from https://advances.sciencemag.org/content/advances/6/3/eaax5145.full.pdf
Tonomura, S., Ebara, S., Bagdasarian, K., Uta, D., Ahissar, E., Meir, I., . . . Kumamoto, K. (2015). Structure-function correlations of rat trigeminal primary neurons: Emphasis on club-like endings, a vibrissal mechanoreceptor. Proceedings of the Japan Academy, Series B, 91(10), 560-576. doi:10.2183/pjab.91.560
Turing, A. (2004). The Chemical Basis of Morphogenesis (1952). In The Chemical Basis of Morphogenesis (1952): Oxford University Press. Retrieved from https://oxford.universitypressscholarship.com/10.1093/oso/9780198250791.001.0001/isbn-9780198250791-book-part-22
Volkov, A. G., Adesina, T., Markin, V. S., & Jovanov, E. (2008). Kinetics and Mechanism of Dionaea muscipula Trap Closing. Plant Physiology, 146(2), 323-324. doi:10.1104/pp.107.108241
Waymaker, L. (n.d.). Hair. In. courses.lumenlearning.com. Retrieved from https://courses.lumenlearning.com/wm-biology2/chapter/hair/